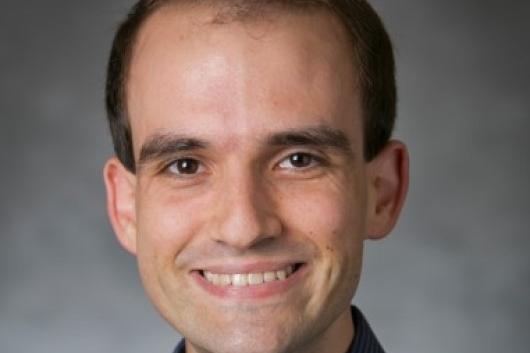
Speaker:
Biostatistics and Bioinformatics Dissertation Defense
Abstract: The generalized score (GS) statistic is widely used to test hypotheses about mean model parameters in the generalized estimating equation (GEE) framework, but in a comparison of predictive values of diagnostic tests or any correlated proportions with unbalanced group sizes, GS has been shown neither to adequately control type I error nor to reduce to the score statistic under independence. Applying weights to the residuals in empirical variance estimation produces a weighted generalized score (WGS) statistic that has been shown to resolve these issues and is now used in the diagnostic testing literature. This dissertation extends the WGS method in several ways. Formulas are derived to adapt the WGS statistic to analyze paired and/or independent data from any exponential family of distributions and with any link function, and the asymptotic convergence of WGS to the chi-square distribution in these general cases is discussed. Finite-sample type I error rates are compared between GS and WGS, for which purpose the variance of the test statistic denominator (i.e., the variance of the empirical variance estimator) is proposed as an analytic heuristic. New weights that optimize the variance-of-the-denominator criterion for approximate type I error control are derived, and simulation results verify that the optimal weights achieve type I error rates closer to the nominal alpha level for combinations of correlation and sample size where either GS or WGS demonstrates poor control.
Zoom Conference: https://zoom.us
Meeting Number: 995 5403 7946
Password: 013499