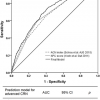
Faculty from the B&B Division of Translational Biomedical Informatics recently published two separate studies on predictive modeling; one in the New England Journal of Medicine (NEJM) and another in the Journal of the American Medical Informatics Association (JAMIA).
The NEJM article was authored by Faculty Michael Pencina, PhD and Ben Goldstein, PhD in collaboration with Ralph B. D’Agostino, PhD, Director of the Biostatistics Core of the Comprehensive Cancer Center at Wake Forest University. In the past decade, U.S. health care systems have amassed large data sets through electronic health records (EHR). This has created an increased demand for prediction models to identify who is at risk for various health conditions. A systematic review revealed weaknesses in model evaluation and highlighted an urgent need for standards for the development, evaluation and application of prediction models. Drs. Pencina, Goldstein and D’Agostino have identified eight key considerations for the introduction and use of prediction models. Read the full article in NEJM here.
The JAMIA article was authored by Faculty Eric Poon, MD in collaboration with other Duke researchers and a current MMCi student. There is little known about how academic medical centers (AMCs) in the US develop, implement, and maintain predictive modeling and machine learning (PM and ML) models. The research team conducted 33 semi-structured interviews with leaders from 19 national AMCs to assess their use of PM and ML in clinical care, understand associated challenges, and determine recommended best practices. Each interview was iteratively coded to identify key barriers to and facilitators of PM and ML adoption and implementation in clinical care. AMCs varied greatly in the use of PM and ML within clinical care, from some just beginning to explore their utility to others with multiple models integrated into clinical care.
Five (5) key barriers to the adoption and implementation of PM and ML were identified in clinical care: (1) culture and personnel, (2) clinical utility of the PM and ML tool, (3) financing, (4) technology, and (5) data. Recommendations to the informatics community to overcome these barriers included: (1) development of robust evaluation methodologies, (2) partnership with vendors, and (3) development and dissemination of best practices. For institutions developing clinical PM and ML applications, they are advised to: (1) develop appropriate governance, (2) strengthen data access, integrity, and provenance, and (3) adhere to the 5 rights of clinical decision support. This article highlights key challenges of implementing PM and ML in clinical care at AMCs and suggests best practices for development, implementation, and maintenance at these institutions. Read the full article in JAMIA here.