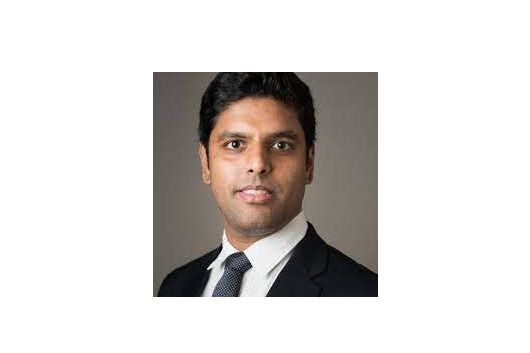
Speaker:
Rishikesan Kamaleswaran, PhD
Abstract: Critically ill patients admitted to the intensive care units face significant challenges due to a combination of deteriorating physiology, and, complex and dynamic care management. A number of measures are abstracted continuously from the patient to understand their potential trajectories, including laboratory measures, physiological data and radiographic imaging. Many of these measures however are constraint by classical problems in selection and measurement biases. Therefore, the key goal of our lab is to utilize observational and irregular temporal data to characterize early identification of abnormal and critical clinical conditions such as severe sepsis, multi-organ dysfunction syndrome and apnea of prematurity. In this talk, I highlight some of the recent works, challenges, and opportunities for characterizing complex and temporally associated interdependencies across multiple organ systems as patients progress through critical illness. We focus on a variety of data driven applications to unravel the complexities of critical illness in order to identify underlying pathobiology and diagnostic markers.
Bio: Dr. Rishikesan Kamaleswaran is an Associate Professor of Biomedical Informatics at Emory University School of Medicine and Biomedical Engineering in the Department of Biomedical Engineering at the Georgia Institute of Technology, Atlanta, GA. He received his PhD in Computer Science from the University of Ontario Institute of Technology in Canada. His areas of interest include machine learning, causal inference, and decision modeling applied to real-time environments in critical and acute care.
Zoom: https://duke.zoom.us/j/93754018275?pwd=OG5HaEljdExFczN0MXNkWUsyRVpvUT09