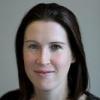
The problem of causal inference from observational data becomes particularly challenging when treatments are initiated over longitudinal follow-up. Robins and colleagues contributed various methods including marginal structural models (MSMs) and g-estimation of structural nested models (SNMs). Matching methods for time-dependent treatments offer a convenient alternative. In essence, the idea is to create pairs of patients, one of whom initiates treatment at time T and another who doesn’t, who are comparable: both eligible for treatment at time T and having a similar covariate history, where that history may include the full, time-dependent information available before time T. This creates a pseudo-experiment for every treated patient. The pairs can be combined to create a matched sample and analyzed using standard methods for matched data. On the surface, this approach produces clinically intuitive results that look just like randomized trials, or observational studies of baseline treatment, including hazard ratios, and survival functions. That is a tremendous advantage, however, there are important complexities in how to define comparable and eligible patients over time. Thus, many variations have been proposed for specific applications, with unique terminology such as sequential stratification matching, propensity score matching with time-dependent covariates, and balanced risk set matching.
A researcher, desiring to understand and apply these methods, will encounter various challenges. Even within the field of biostatistics and epidemiology, related methods use distinct jargon so they are not easily found by searching. In addition, the existing articles are framed around solving a specific problem, and not in terms of generalizable principles and alternatives. Aim 1 will address this gap by conducting a comprehensive review of longitudinal matching methods in order to identify the relevant alternatives, advantages, disadvantages and choices that should be considered explicitly in all longitudinal matched analyses.
Statistical methods for time-dependent confounding are under-utilized and poorly appreciated in clinical research. This gap is exemplified by the fact that propensity score matching with time-dependent covariates has only been referenced in one cardiovascular publication that did not apply the method, but recommended it for future studies. Similarly, a rigorous statistical paper on sequential stratification matching appeared in the Journal of the American Statistical Association in 2009, but does not appear to have been referenced beyond studies conducted by the original authors, colleagues and ourselves. Perhaps the most powerful way to promote the use of statistical methods is to show that they matter in real applications. In Aim 2, we will use the data sources available at Duke Clinical Research Institute, such as the Framingham Heart Study, to assess the impact of longitudinal matching methods when compared to naïve methods and alternative strategies for time-dependent confounding.